实例
数据集
笔记本
笔记本

DINO 自监督算法简介
DINO 的初衷是质疑自监督学习相较于 CNN 是否为 Transformer(ViT) 提供了新的属性,但是在过程中,发现:
- 自监督 ViT 的特征在图像的语义分割中包含的显式信息,这是之前监督学习下无论 ViT 还是 CNN 都没有出现的情况。
- 这个架构用一个很小的 ViT 提取出来的特征用 k-NN 来对 ImageNet 图像数据集进行分类可以达到 78.3% top-1
论文将这个自监督结构命名为 DINO 🦖 ,这种没有引入标签的“自蒸馏”形式在结合各种 ViTs 以后可以达到 80.1% top-1.
笔记本内容
Emerging Properties in Self-Supervised Vision Transformers #
DINO 的初衷是质疑自监督学习相较于 CNN 是否为 Transformer(ViT) 提供了新的属性,但是在过程中,发现:
- 自监督 ViT 的特征在图像的语义分割中包含的显式信息,这是之前监督学习下无论 ViT 还是 CNN 都没有出现的情况。
- 这个架构用一个很小的 ViT 提取出来的特征用 k-NN 来对 ImageNet 图像数据集进行分类可以达到 78.3% top-1
论文将这个自监督结构命名为 DINO 🦖 ,这种没有引入标签的“自蒸馏”形式在结合各种 ViTs 以后可以达到 80.1% top-1.
下面的这幅 GIF 很简洁地表示了 DINO 的结构:
DINO 的结构 #
其中 Student 模型和 Teacher 模型是完全一样的
在训练中,Teacher 模型是没有训练的,它的权重更新是通过对 Student 模型权重的 EMA(exponential moving average ) 来更新 Teacher 模型的。下面的图是 EMA 的举例,可以感受一下。
还有值得注意的是两个模型的输入是完全独立的数据增强。
具体的表示可以参考 [Ref: https://en.wikipedia.org/wiki/Moving_average]
Pseudocode #
下载源码 #
下面就是代码层面的一些展示了。
!git clone https://github.com/facebookresearch/dino.git /home/featurize/dino
Cloning into '/home/featurize/dino'... remote: Enumerating objects: 168, done.[K remote: Counting objects: 100% (24/24), done.[K remote: Compressing objects: 100% (19/19), done.[K remote: Total 168 (delta 10), reused 16 (delta 5), pack-reused 144[K Receiving objects: 100% (168/168), 24.47 MiB | 10.41 MiB/s, done. Resolving deltas: 100% (98/98), done.
## 目录准备以及下载图片样例
!mkdir /home/featurize/dino/input
!mkdir /home/featurize/dino/output
!wget https://www.snowskool.com/uploads/images/ski_and_snowboard_snowsport12.jpg -O /home/featurize/dino/input/ski.jpg
import matplotlib.pyplot as plt
import cv2
plt.axis('off')
plt.imshow(cv2.cvtColor(cv2.imread('/home/featurize/dino/input/ski.jpg'), cv2.COLOR_BGR2RGB));
mkdir: cannot create directory ‘/home/featurize/dino/input’: File exists mkdir: cannot create directory ‘/home/featurize/dino/output’: File exists --2021-11-30 14:38:32-- https://www.snowskool.com/uploads/images/ski_and_snowboard_snowsport12.jpg Connecting to 172.16.0.13:7890... connected. Proxy request sent, awaiting response... 200 OK Length: 110231 (108K) [image/jpeg] Saving to: ‘/home/featurize/dino/input/ski.jpg’ /home/featurize/din 100%[===================>] 107.65K 347KB/s in 0.3s 2021-11-30 14:38:34 (347 KB/s) - ‘/home/featurize/dino/input/ski.jpg’ saved [110231/110231]
对图片进行推理 #
!python /home/featurize/dino/visualize_attention.py \
--image_path /home/featurize/dino/input/ski.jpg \
--output_dir /home/featurize/dino/output
Please use the `--pretrained_weights` argument to indicate the path of the checkpoint to evaluate. Since no pretrained weights have been provided, we load the reference pretrained DINO weights. Downloading: "https://dl.fbaipublicfiles.com/dino/dino_deitsmall8_300ep_pretrain/dino_deitsmall8_300ep_pretrain.pth" to /home/featurize/.cache/torch/hub/checkpoints/dino_deitsmall8_300ep_pretrain.pth 100%|██████████████████████████████████████| 82.7M/82.7M [00:08<00:00, 10.4MB/s] /environment/python/versions/3.7.12/lib/python3.7/site-packages/torch/nn/functional.py:3635: UserWarning: Default upsampling behavior when mode=bicubic is changed to align_corners=False since 0.4.0. Please specify align_corners=True if the old behavior is desired. See the documentation of nn.Upsample for details. "See the documentation of nn.Upsample for details.".format(mode) /environment/python/versions/3.7.12/lib/python3.7/site-packages/torch/nn/functional.py:3680: UserWarning: The default behavior for interpolate/upsample with float scale_factor changed in 1.6.0 to align with other frameworks/libraries, and now uses scale_factor directly, instead of relying on the computed output size. If you wish to restore the old behavior, please set recompute_scale_factor=True. See the documentation of nn.Upsample for details. "The default behavior for interpolate/upsample with float scale_factor changed " /home/featurize/dino/output/attn-head0.png saved. /home/featurize/dino/output/attn-head1.png saved. /home/featurize/dino/output/attn-head2.png saved. /home/featurize/dino/output/attn-head3.png saved. /home/featurize/dino/output/attn-head4.png saved. /home/featurize/dino/output/attn-head5.png saved.
f, axs = plt.subplots(2,3, figsize=(12,8))
for i, ax in enumerate(axs.reshape(-1)):
ax.axis('off')
ax.imshow(cv2.cvtColor(cv2.imread(f'/home/featurize/dino/output/attn-head{i}.png'), cv2.COLOR_BGR2RGB));
下面试一下视频 #
# 下载测试视频
!unset http_proxy;unset https_proxy;unset all_proxy
!wget https://featurize.oss-cn-chengdu.aliyuncs.com/input.mp4 -O /home/featurize/dino/input/input.mp4
# Jupyter里可视化一下
from IPython.display import Video
Video('/home/featurize/dino/input/input.mp4', embed=True, width=800, height=600)
# DINO 进行推理
!python /home/featurize/dino/video_generation.py \
--pretrained_weights dino_deitsmall8_pretrain.pth \
--input_path /home/featurize/dino/input/input.mp4 \
--output_path /home/featurize/dino/output \
--video_format mp4 \
--fps 25
Please use the `--pretrained_weights` argument to indicate the path of the checkpoint to evaluate. Since no pretrained weights have been provided, we load the reference pretrained DINO weights. Video: /home/featurize/dino/input/input.mp4 (24.978432290124694 fps) Extracting frames to /home/featurize/dino/output/frames Generating attention images to /home/featurize/dino/output/attention 0%| | 0/613 [00:00<?, ?it/s]/environment/python/versions/3.7.12/lib/python3.7/site-packages/torch/nn/functional.py:3635: UserWarning: Default upsampling behavior when mode=bicubic is changed to align_corners=False since 0.4.0. Please specify align_corners=True if the old behavior is desired. See the documentation of nn.Upsample for details. "See the documentation of nn.Upsample for details.".format(mode) /environment/python/versions/3.7.12/lib/python3.7/site-packages/torch/nn/functional.py:3680: UserWarning: The default behavior for interpolate/upsample with float scale_factor changed in 1.6.0 to align with other frameworks/libraries, and now uses scale_factor directly, instead of relying on the computed output size. If you wish to restore the old behavior, please set recompute_scale_factor=True. See the documentation of nn.Upsample for details. "The default behavior for interpolate/upsample with float scale_factor changed " 100%|█████████████████████████████████████████| 613/613 [11:57<00:00, 1.17s/it] Generating video (1280, 720) to /home/featurize/dino/output 100%|█████████████████████████████████████████| 612/612 [00:07<00:00, 85.13it/s] OpenCV: FFMPEG: tag 0x5634504d/'MP4V' is not supported with codec id 12 and format 'mp4 / MP4 (MPEG-4 Part 14)' OpenCV: FFMPEG: fallback to use tag 0x7634706d/'mp4v' Done
# 这是 Dave 之前推理的视频
Video('https://featurize.oss-cn-chengdu.aliyuncs.com/output.mp4')
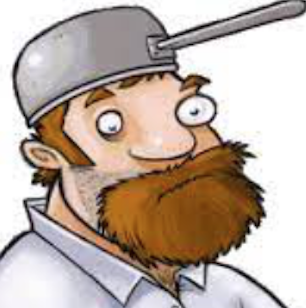
Dave 觉得自监督学习在并未输入人类的理解信息的情况下,模型能够给出语义级别的解释并且能够超过监督学习,这就十分符合我对智能体的期望。
评论(0条)